Overlooked Principles in AI Medical Device Development: A Guide for Startups
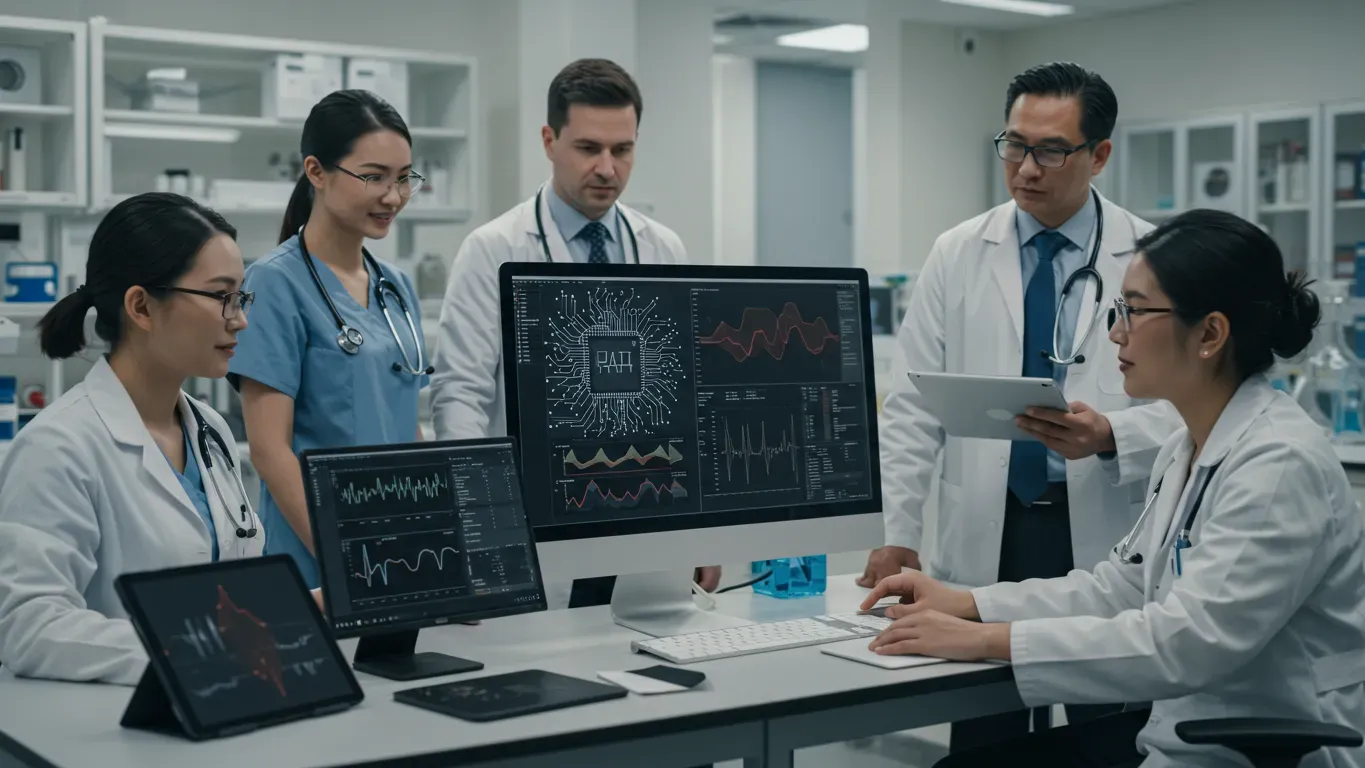
The intersection of artificial intelligence (AI) and healthcare is revolutionizing how medical devices are designed, developed, and deployed. However, with rapid advancements come unique challenges—especially for startups navigating this complex landscape. The FDA guidelines on Good Machine Learning Practice for Medical Device Development: Guiding Principles is a great source of information for any startup or company looking into developing AI medical devices to overcome these challenges. However, while many companies focus on cutting-edge algorithms and innovative applications, they often overlook critical principles that ensure safety, efficacy, and long-term success.
This blog post highlights three key principles out of the 10 principles mentioned in the FDA guidelines. In my experience, these three principles are frequently underestimated by startups developing AI medical devices: multi-disciplinary expertise, representative clinical data sets, and monitoring deployed models. By understanding and prioritizing these areas, companies can build more robust, equitable, and reliable AI solutions.
1. Leverage Multi-Disciplinary Expertise Throughout the Product Lifecycle
AI medical devices are not standalone technologies—they integrate into complex clinical workflows and impact patient care directly. To ensure that these devices meet clinical needs, mitigate risks, and deliver meaningful benefits, it is essential to involve a diverse range of experts throughout the development process.
Why This Principle Is Overlooked:
Startups often rely on small teams with overlapping roles, which can lead to knowledge gaps. For example, engineers may dominate the design phase, while clinicians or ethicists are only consulted later in the process. This siloed approach risks creating devices that do not align with real-world clinical needs or fail to address critical patient safety concerns.
How Startups Can Implement This:
- Build a Multi-Disciplinary Team: Include data scientists, software engineers, clinicians, ethicists, and regulatory experts from the outset. Each member brings unique perspectives that contribute to a holistic understanding of the product’s intended use.
- Engage Stakeholders Early: Collaborate with healthcare providers, patients, and regulators during the design phase to ensure the device integrates seamlessly into clinical workflows.
- Foster Continuous Learning: Encourage cross-functional training and knowledge sharing to bridge gaps in expertise.
By embracing multi-disciplinary collaboration, startups can develop AI medical devices that are not only technically sound but also clinically relevant and user-friendly.
2. Ensure Clinical Study Participants and Data Sets Are Representative
AI models are only as good as the data they’re trained on. For medical devices, this means ensuring that training and test datasets reflect the diversity of the intended patient population. If a model is developed using biased or non-representative data, it may perform poorly for certain groups, leading to inequitable outcomes.
Why This Principle Is Overlooked:
Startups often prioritize speed over thoroughness, which can result in shortcuts during data collection. For example, they may rely on readily available datasets that lack diversity or fail to account for factors like age, sex, race, and ethnicity. This oversight can have serious consequences, such as underperformance for marginalized patient groups.
How Startups Can Implement This:
- Define the Intended Patient Population Early: Clearly articulate who will benefit from the device and ensure that clinical studies include a diverse sample of patients.
- Collect High-Quality Data: Invest in robust data collection protocols that minimize bias and ensure data integrity. Partner with diverse healthcare providers to gather representative samples.
- Conduct Bias Assessments: Perform regular audits to identify and mitigate biases in datasets and model outputs.
By prioritizing representativeness, startups can develop AI medical devices that are equitable and effective for all patients.
3. Monitor Deployed Models and Manage Re-Training Risks
AI models are not static—they evolve over time as they encounter new data in real-world settings. However, this evolution can introduce risks such as performance degradation, unintended bias, or security vulnerabilities if not properly managed.
Why This Principle Is Overlooked:
Startups often focus on launching their product rather than planning for long-term monitoring and maintenance. They may assume that the model will remain stable after deployment, ignoring the need for continuous oversight and adaptation.
How Startups Can Implement This:
- Establish Monitoring Systems: Implement tools to track model performance in real-world conditions, including metrics like accuracy, safety, and user satisfaction.
- Plan for Updates and Re-Training: Develop protocols for re-training models with new data while minimizing risks like overfitting or bias. Ensure that updates are transparently documented and communicated to users.
- Engage Regulatory Authorities: Work closely with regulators to ensure ongoing compliance with evolving standards for AI medical devices.
By monitoring deployed models and managing re-training risks, startups can ensure their AI solutions remain safe, effective, and reliable over time.
Conclusion
The development of AI medical devices is a complex endeavor that requires careful attention to both technical and clinical considerations. Startups often overlook critical principles like multi-disciplinary expertise, representative data sets, and model monitoring—yet these are essential for building trustworthy, equitable, and high-quality solutions.
By prioritizing these principles from the outset, startups can navigate the challenges of AI development more effectively and deliver devices that truly transform healthcare. The journey may be challenging, but the rewards—improved patient outcomes and lasting innovation—are well worth the effort.
Let’s build AI medical devices that not only innovate but also inspire confidence in patients and clinicians alike.